Training with multiple TPU cores is incorrect and slower
See original GitHub issueSystem Info
- `Accelerate` version: 0.10.0
- Platform: Linux-5.13.0-1023-gcp-x86_64-with-glibc2.29
- Python version: 3.8.10
- Numpy version: 1.22.4
- PyTorch version (GPU?): 1.11.0a0+gitbc2c6ed (False)
- `Accelerate` default config:
- compute_environment: LOCAL_MACHINE
- distributed_type: TPU
- mixed_precision: no
- use_cpu: False
- num_processes: 8
- machine_rank: 0
- num_machines: 1
- main_process_ip: None
- main_process_port: None
- main_training_function: main
- deepspeed_config: {}
- fsdp_config: {}
Information
- The official example scripts
- My own modified scripts
Tasks
- One of the scripts in the examples/ folder of Accelerate or an officially supported
no_trainer
script in theexamples
folder of thetransformers
repo (such asrun_no_trainer_glue.py
) - My own task or dataset (give details below)
Reproduction
First, train with 8 TPU cores (run the script twice and count the second one to exclude the time used to download the datasets)
$ pipenv run accelerate config
In which compute environment are you running? ([0] This machine, [1] AWS (Amazon SageMaker)): 0
Which type of machine are you using? ([0] No distributed training, [1] multi-CPU, [2] multi-GPU, [3] TPU): 3
What is the name of the function in your script that should be launched in all parallel scripts? [main]:
How many TPU cores should be used for distributed training? [1]:8
$ time pipenv run accelerate launch accelerate/examples/nlp_example.py
...a lot of irrelevant warnings...
epoch 0: {'accuracy': 0.3125, 'f1': 0.0}
epoch 1: {'accuracy': 0.671875, 'f1': 0.8004750593824228}
epoch 2: {'accuracy': 0.6796875, 'f1': 0.8079625292740047}
________________________________________________________
Executed in 157.17 secs fish external
usr time 474.51 secs 592.00 micros 474.51 secs
sys time 99.76 secs 0.00 micros 99.76 secs
Next, train with 1 TPU core
$ pipenv run accelerate config
In which compute environment are you running? ([0] This machine, [1] AWS (Amazon SageMaker)): 0
Which type of machine are you using? ([0] No distributed training, [1] multi-CPU, [2] multi-GPU, [3] TPU): 3
What is the name of the function in your script that should be launched in all parallel scripts? [main]:
How many TPU cores should be used for distributed training? [1]:1
$ time pipenv run accelerate launch accelerate/examples/nlp_example.py
...a lot of irrelevant warnings...
epoch 0: {'accuracy': 0.6838235294117647, 'f1': 0.8122270742358079}
epoch 1: {'accuracy': 0.7205882352941176, 'f1': 0.8256880733944955}
epoch 2: {'accuracy': 0.7156862745098039, 'f1': 0.8215384615384616}
________________________________________________________
Executed in 150.81 secs fish external
usr time 352.98 secs 419.00 micros 352.98 secs
sys time 34.38 secs 210.00 micros 34.38 secs
Expected behavior
+ The `accuracy` and `f1` should be very close, but they are way off, and things are particularly weird in epoch 0 when we use 8 cores.
+ Using 8 TPU cores should be much faster, but it's slower (157.17s vs 150.81s).
Issue Analytics
- State:
- Created a year ago
- Comments:8 (1 by maintainers)
Top Results From Across the Web
Troubleshooting TensorFlow - TPU - Google Cloud
This message can occur when training with TensorFlow, PyTorch, or JAX. Details. Each Cloud TPU is made of eight TPU cores, v2 TPUs...
Read more >Training Large-Scale Recommendation Models with TPUs
TPUs can offer much faster training speed and significantly lower training costs for recommendation system models than the CPUs.
Read more >Why is Google Colab TPU as slow as my computer?
Since I have a large dataset and not much power in my PC, I thought it was a good idea to use TPU...
Read more >Hugging Face on PyTorch / XLA TPUs
In a typical XLA:TPU training scenario we're training on multiple TPU cores in parallel (a single Cloud TPU device includes 8 TPU cores)....
Read more >Tensor Processing Unit (TPU) - PyTorch Lightning
This error is raised when the XLA device is called outside the spawn process. Internally in TPUSpawn Strategy for training on multiple tpu...
Read more >
Top Related Medium Post
No results found
Top Related StackOverflow Question
No results found
Troubleshoot Live Code
Lightrun enables developers to add logs, metrics and snapshots to live code - no restarts or redeploys required.
Start Free
Top Related Reddit Thread
No results found
Top Related Hackernoon Post
No results found
Top Related Tweet
No results found
Top Related Dev.to Post
No results found
Top Related Hashnode Post
No results found
@nalzok the bug is indeed with the NLP example script, I’ll put in a fix tommorow. What I did here was took the NLP Example notebook (found here), put it into a script, and I could get the right training results on 8 cores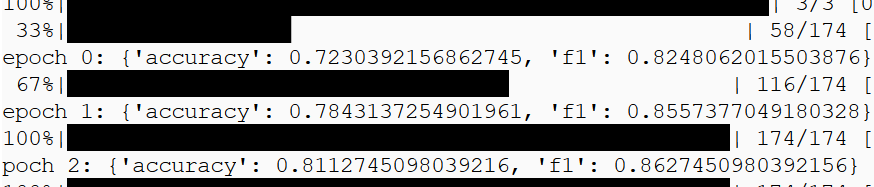
Thanks for your time!
To clarify, I am on a TPU v3-8 machine on GCP (obtained through TRC), not Colab. Additionally, the architecture is a TPU VM instead of a TPU node, so there are no networking roundtrips (i.e.
$XRT_TPU_CONFIG
islocalservice;0;localhost:51011
).