Ability to fine-tune whisper large on a GPU with 24 gb of ram
See original GitHub issueFeature request
I’ve been trying to fine-tune whisper large on a GPU with 24gb of ram (both single GPU and multi GPU) and I run out of memory while training (with batch size set to 1 and max-length of audio set to 2.5 seconds).
I made this a feature request not a bug report since I don’t believe there is a problem with the code.
Training script
Training code
from datasets import load_dataset, DatasetDict
common_voice = DatasetDict()
#common_voice["train"] = load_dataset("mozilla-foundation/common_voice_11_0", "sv-SE", split="train+validation", use_auth_token=True)
#common_voice["test"] = load_dataset("mozilla-foundation/common_voice_11_0", "sv-SE", split="test", use_auth_token=True)
common_voice["train"] = load_dataset("mozilla-foundation/common_voice_11_0", "sv-SE", split="train[:1%]+validation[:1%]", use_auth_token=True)
common_voice["test"] = load_dataset("mozilla-foundation/common_voice_11_0", "sv-SE", split="test[:1%]", use_auth_token=True)
print(common_voice)
common_voice = common_voice.remove_columns(["accent", "age", "client_id", "down_votes", "gender", "locale", "path", "segment", "up_votes"])
print(common_voice)
from transformers import WhisperFeatureExtractor
feature_extractor = WhisperFeatureExtractor.from_pretrained("openai/whisper-large")
from transformers import WhisperTokenizer
tokenizer = WhisperTokenizer.from_pretrained("openai/whisper-large", language="swedish", task="transcribe")
from transformers import WhisperProcessor
processor = WhisperProcessor.from_pretrained("openai/whisper-large", language="swedish", task="transcribe")
print(common_voice["train"][0])
from datasets import Audio
common_voice = common_voice.cast_column("audio", Audio(sampling_rate=16000))
common_voice = common_voice.filter(lambda example: len(example["audio"]["array"]) < 2.5 * 16000, load_from_cache_file=False)
print(common_voice["train"][0])
def prepare_dataset(batch):
# load and resample audio data from 48 to 16kHz
audio = batch["audio"]
# compute log-Mel input features from input audio array
batch["input_features"] = feature_extractor(audio["array"], sampling_rate=audio["sampling_rate"]).input_features[0]
# encode target text to label ids
batch["labels"] = tokenizer(batch["sentence"]).input_ids
return batch
common_voice = common_voice.map(prepare_dataset, remove_columns=common_voice.column_names["train"], num_proc=1)
import torch
from dataclasses import dataclass
from typing import Any, Dict, List, Union
@dataclass
class DataCollatorSpeechSeq2SeqWithPadding:
processor: Any
def __call__(self, features: List[Dict[str, Union[List[int], torch.Tensor]]]) -> Dict[str, torch.Tensor]:
# split inputs and labels since they have to be of different lengths and need different padding methods
# first treat the audio inputs by simply returning torch tensors
input_features = [{"input_features": feature["input_features"]} for feature in features]
batch = self.processor.feature_extractor.pad(input_features, return_tensors="pt")
# get the tokenized label sequences
label_features = [{"input_ids": feature["labels"]} for feature in features]
# pad the labels to max length
labels_batch = self.processor.tokenizer.pad(label_features, return_tensors="pt")
# replace padding with -100 to ignore loss correctly
labels = labels_batch["input_ids"].masked_fill(labels_batch.attention_mask.ne(1), -100)
# if bos token is appended in previous tokenization step,
# cut bos token here as it's append later anyways
if (labels[:, 0] == self.processor.tokenizer.bos_token_id).all().cpu().item():
labels = labels[:, 1:]
batch["labels"] = labels
return batch
"""Let's initialise the data collator we've just defined:"""
data_collator = DataCollatorSpeechSeq2SeqWithPadding(processor=processor)
import evaluate
metric = evaluate.load("wer")
def compute_metrics(pred):
pred_ids = pred.predictions
label_ids = pred.label_ids
# replace -100 with the pad_token_id
label_ids[label_ids == -100] = tokenizer.pad_token_id
# we do not want to group tokens when computing the metrics
pred_str = tokenizer.batch_decode(pred_ids, skip_special_tokens=True)
label_str = tokenizer.batch_decode(label_ids, skip_special_tokens=True)
wer = 100 * metric.compute(predictions=pred_str, references=label_str)
return {"wer": wer}
from transformers import WhisperForConditionalGeneration
model = WhisperForConditionalGeneration.from_pretrained("openai/whisper-large")
model.config.forced_decoder_ids = None
model.config.suppress_tokens = []
from transformers import Seq2SeqTrainingArguments
training_args = Seq2SeqTrainingArguments(
output_dir="./whisper-large-sv-test2", # change to a repo name of your choice
per_device_train_batch_size=1,
gradient_accumulation_steps=1, # increase by 2x for every 2x decrease in batch size
learning_rate=1e-5,
warmup_steps=1,
max_steps=10,
gradient_checkpointing=True,
fp16=True,
group_by_length=True,
evaluation_strategy="steps",
per_device_eval_batch_size=1,
predict_with_generate=True,
generation_max_length=225,
save_steps=5, # set to < max_steps
eval_steps=5, # set to < max_steps
logging_steps=1, # set to < max_steps
report_to=["tensorboard"],
load_best_model_at_end=True,
metric_for_best_model="wer",
greater_is_better=False,
push_to_hub=True,
)
from transformers import Seq2SeqTrainer
trainer = Seq2SeqTrainer(
args=training_args,
model=model,
train_dataset=common_voice["train"],
eval_dataset=common_voice["test"],
data_collator=data_collator,
compute_metrics=compute_metrics,
tokenizer=processor.feature_extractor,
)
processor.save_pretrained(training_args.output_dir)
trainer.train()
kwargs = {
"dataset_tags": "mozilla-foundation/common_voice_11_0",
"dataset": "Common Voice 11.0", # a 'pretty' name for the training dataset
"language": "sv",
"model_name": "whisper-large-sv-test2", # a 'pretty' name for our model
"finetuned_from": "openai/whisper-large",
"tasks": "automatic-speech-recognition",
"tags": "hf-asr-leaderboard",
}
trainer.push_to_hub(**kwargs)
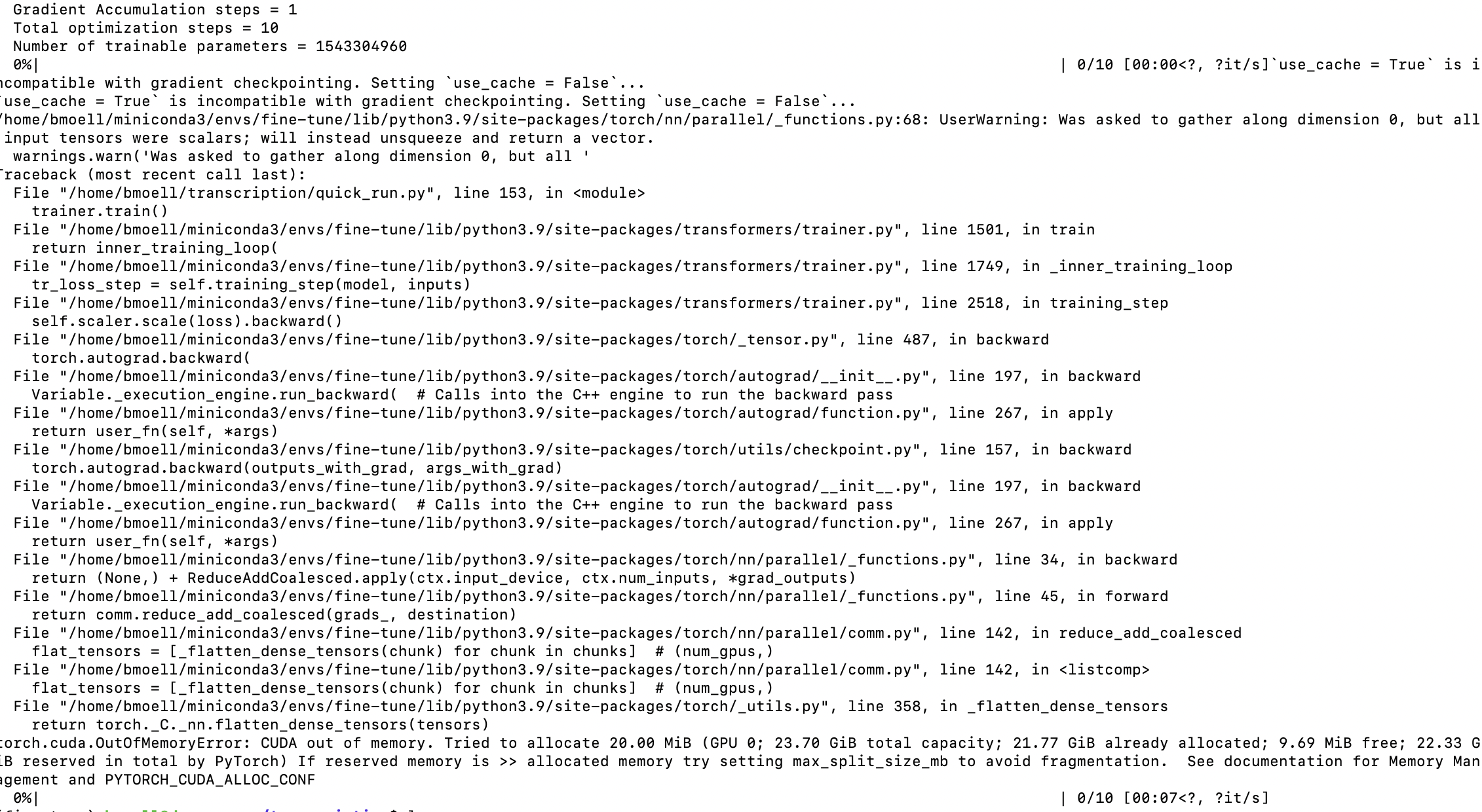
Motivation
It would be great if it would be able to fine-tune the large model on a 24gb GPU since that would make it much more easy to train the larger mode…
Your contribution
I would love to help out with this issue.
Issue Analytics
- State:
- Created 10 months ago
- Comments:11 (8 by maintainers)
Top Results From Across the Web
Memory requirements? #5 - openai/whisper - GitHub
I attempted to run whisper on an audio file using the medium model, and I got this: ... Model, Time, s, CPU/GPU, RAM,...
Read more >[D] Some OpenAI Whisper benchmarks for runtime and cost
I ran a few benchmarks on Whisper's runtime and cost-to-run on GCP, so just dropping it here in case it's valuable to anyone!...
Read more >Whisper – open source speech recognition by OpenAI
Having this fully open is a big deal though - now that level of transcription ability can be wrapped as an audio plugin...
Read more >24gb vs 16gb : Mixing RAM capacity (FPS test) - YouTube
Does mixing different RAM sticks affect gaming performance ? Tested with 8gb + 8gb vs. 16gb + 8gb. Test detailsTested at 1080p resolution....
Read more >Deep Learning GPU with 24GB RAM for less than 350
Blog: https://pysource.com/2022/02/08/the-best-deep-learning- gpu -for-less-than-350- nvidia -tesla-k80/Today we will see a video card with 24GB ...
Read more >
Top Related Medium Post
No results found
Top Related StackOverflow Question
No results found
Troubleshoot Live Code
Lightrun enables developers to add logs, metrics and snapshots to live code - no restarts or redeploys required.
Start Free
Top Related Reddit Thread
No results found
Top Related Hackernoon Post
No results found
Top Related Tweet
No results found
Top Related Dev.to Post
No results found
Top Related Hashnode Post
No results found
cc @younesbelkada the 8bit master
In general though, the 8bit model will be slower. Hence the suggestion for changing the optimiser first.
Hey @BirgerMoell - thanks for opening this feature request and for your interest in the Whisper model 🗣🇸🇪 I’ve made the code in your original post a drop-down for ease of reading.
The examples script run_speech_recognition_seq2seq.py has recently been updated to handle Whisper (https://github.com/huggingface/transformers/pull/19519), so you can use this as an end-to-end script for training your system! All you have to do is modify the example training config given in the README for your language of choice: examples/pytorch/speech-recognition#whisper-model And then execute the command! The rest will be taken care for you 🤗
A couple of things:
per_device_batch_size=2
andgradient_accumulation_steps=16
). There are some things we can try to make the model / training more memory efficient if you want to use the medium or large checkpoints! (see below)Now, assuming that you do want to train a bigger model than the ‘small’ checkpoint, you can either try the training script with the medium checkpoint and a
per_device_batch_size
of 2 or 4, or you can try using the large checkpoint with some memory hacks:and then set
optim="adamw_bnb_8bit"
when you instantiate theSeq2SeqTrainingArguments
:Check out the docs for more details: (https://huggingface.co/docs/transformers/main_classes/trainer#transformers.Seq2SeqTrainingArguments.optim)
optim="adafactor"
. This is untested for fine-tuning Whisper, so I’m not sure how Adafactor performance compares to Adam.Neither 1 or 2 are tested, so I can’t guarantee that they’ll work, but they’re easy approaches to try! One line code changes for each. I’d try 1 first then 2, as there shouldn’t be a performance degradation trying 1, but there might be with 2.
I’ll reiterate again that the medium checkpoint is a good option for a device < 80GB memory!