CUDA out of memory
See original GitHub issueI get a OOM when loading the upsample
model:
options_up = model_and_diffusion_defaults_upsampler()
options_up['use_fp16'] = has_cuda
options_up['timestep_respacing'] = 'fast27' # use 27 diffusion steps for very fast sampling
model_up, diffusion_up = create_model_and_diffusion(**options_up)
model_up.eval()
if has_cuda:
model_up.convert_to_fp16()
model_up.to(device)
model_up.load_state_dict(load_checkpoint('upsample', device))
print('total upsampler parameters', sum(x.numel() for x in model_up.parameters()))
the allocation error was
RuntimeError: CUDA out of memory. Tried to allocate 100.00 MiB (GPU 0; 3.94 GiB total capacity; 3.00 GiB already allocated; 30.94 MiB free; 3.06 GiB reserved in total by PyTorch) If reserved memory is >> allocated memory try setting max_split_size_mb to avoid fragmentation. See documentation for Memory Management and PYTORCH_CUDA_ALLOC_CONF
mynvidia-smi
is
loreto@ombromanto:~/Projects/glide-text2im$ nvidia-smi
Wed Dec 22 20:39:15 2021
+-----------------------------------------------------------------------------+
| NVIDIA-SMI 460.32.03 Driver Version: 460.32.03 CUDA Version: 11.2 |
|-------------------------------+----------------------+----------------------+
| GPU Name Persistence-M| Bus-Id Disp.A | Volatile Uncorr. ECC |
| Fan Temp Perf Pwr:Usage/Cap| Memory-Usage | GPU-Util Compute M. |
| | | MIG M. |
|===============================+======================+======================|
| 0 GeForce GTX 105... Off | 00000000:01:00.0 On | N/A |
| 45% 23C P5 N/A / 75W | 3994MiB / 4033MiB | 2% Default |
| | | N/A |
+-------------------------------+----------------------+----------------------+
+-----------------------------------------------------------------------------+
| Processes: |
| GPU GI CI PID Type Process name GPU Memory |
| ID ID Usage |
|=============================================================================|
| 0 N/A N/A 1094 G /usr/lib/xorg/Xorg 121MiB |
| 0 N/A N/A 1926 G /usr/bin/gnome-shell 26MiB |
| 0 N/A N/A 3532 G ...AAAAAAAA== --shared-files 22MiB |
| 0 N/A N/A 4795 C /usr/bin/python 3819MiB |
+-----------------------------------------------------------------------------+
Issue Analytics
- State:
- Created 2 years ago
- Comments:8
Top Results From Across the Web
"RuntimeError: CUDA error: out of memory" - Stack Overflow
The error occurs because you ran out of memory on your GPU. One way to solve it is to reduce the batch size...
Read more >Solving "CUDA out of memory" Error - Kaggle
Solving "CUDA out of memory" Error · 1) Use this code to see memory usage (it requires internet to install package): · 2)...
Read more >Solving the “RuntimeError: CUDA Out of memory” error
Solving the “RuntimeError: CUDA Out of memory” error · Reduce the `batch_size` · Lower the Precision · Do what the error says ·...
Read more >Resolving CUDA Being Out of Memory With Gradient ...
Implementing gradient accumulation and automatic mixed precision to solve CUDA out of memory issue when training big deep learning models ...
Read more >Stable Diffusion Runtime Error: How To Fix CUDA Out Of ...
How To Fix Runtime Error: CUDA Out Of Memory In Stable Diffusion · Restarting the PC worked for some people. · Reduce the...
Read more >
Top Related Medium Post
No results found
Top Related StackOverflow Question
No results found
Troubleshoot Live Code
Lightrun enables developers to add logs, metrics and snapshots to live code - no restarts or redeploys required.
Start Free
Top Related Reddit Thread
No results found
Top Related Hackernoon Post
No results found
Top Related Tweet
No results found
Top Related Dev.to Post
No results found
Top Related Hashnode Post
No results found
Amazing, thanks! In fact I read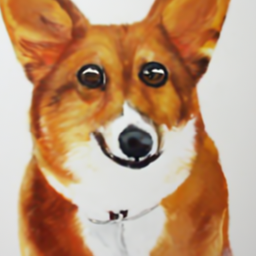
total upsampler parameters 398361286
. Using the CPU trick it worked with the 4GB GTX 1050. Also it took few seconds to generate this curious dogMaybe this approach could be a guideline in the docs…
I haven’t tested this code with less than 16GB of GPU memory, but this is a bit surprising since each model is roughly 400M parameters and therefore around 800MB of memory.
One suggestion: try loading the checkpoint on CPU, and then moving to GPU, like so: