Reconsider default of `max_features` of RandomForestRegressor
See original GitHub issueIn https://github.com/scikit-learn/scikit-learn/issues/7254, there was a long discussion on max_features
defaults for random forests. As a consequence, the default “auto” was changed to “sqrt” for RandomForestClassifier, but unfortunately not for RandomForestRegressor. I would like to reconsider this decision.
What to change?
The default of RandomForestRegressor’s max_features = "auto"
should point to m/3 or sqrt(m), where m is the number of features.
Why?
-
Good defaults are essential for random forests. The fact that random forests do well even without hyperparameter tuning is one of their only advantages over boosted trees.
-
Every implementation in R and also h2o use sqrt(m) or m/3 as default. R’s
ranger
package uses sqrt(m) for both regression and classification. https://github.com/imbs-hl/ranger -
Column subsampling per split is the main source of randomness, leading to less correlated trees. The current default removes this effect. Strictly speaking, the current default does not fit a proper random forest but rather a bagged tree. My experience shows that random forests perform better than bagged trees in the majority of the cases.
-
Training time is proportional to
max_features
. I.e. one could easily run 500 trees instead of 100 with a better default.
Note: I am not talking about defaults for completely randomized trees, just about proper random forests.
Issue Analytics
- State:
- Created 2 years ago
- Reactions:1
- Comments:34 (31 by maintainers)
Curious about the effects of
max_features
in regression problems, I did an analysis (thanks @thomasjpfan for your sk_encoder_cv repo!), see https://github.com/lorentzenchr/notebooks/blob/master/random_forests_max_features.ipynb.5-fold CV: MSE and uncertainty (std)
Smaller is better.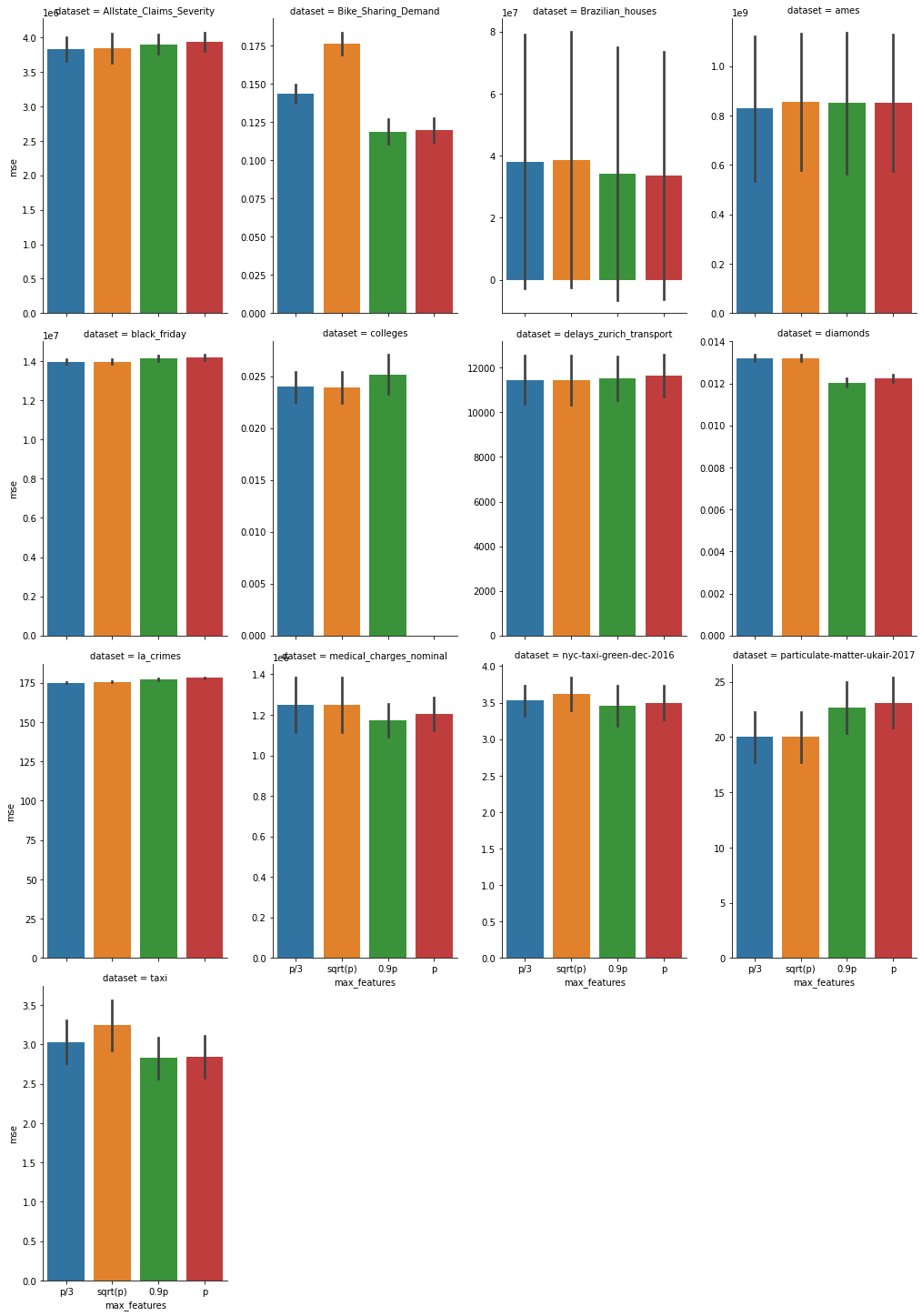
Fit times
Smaller is better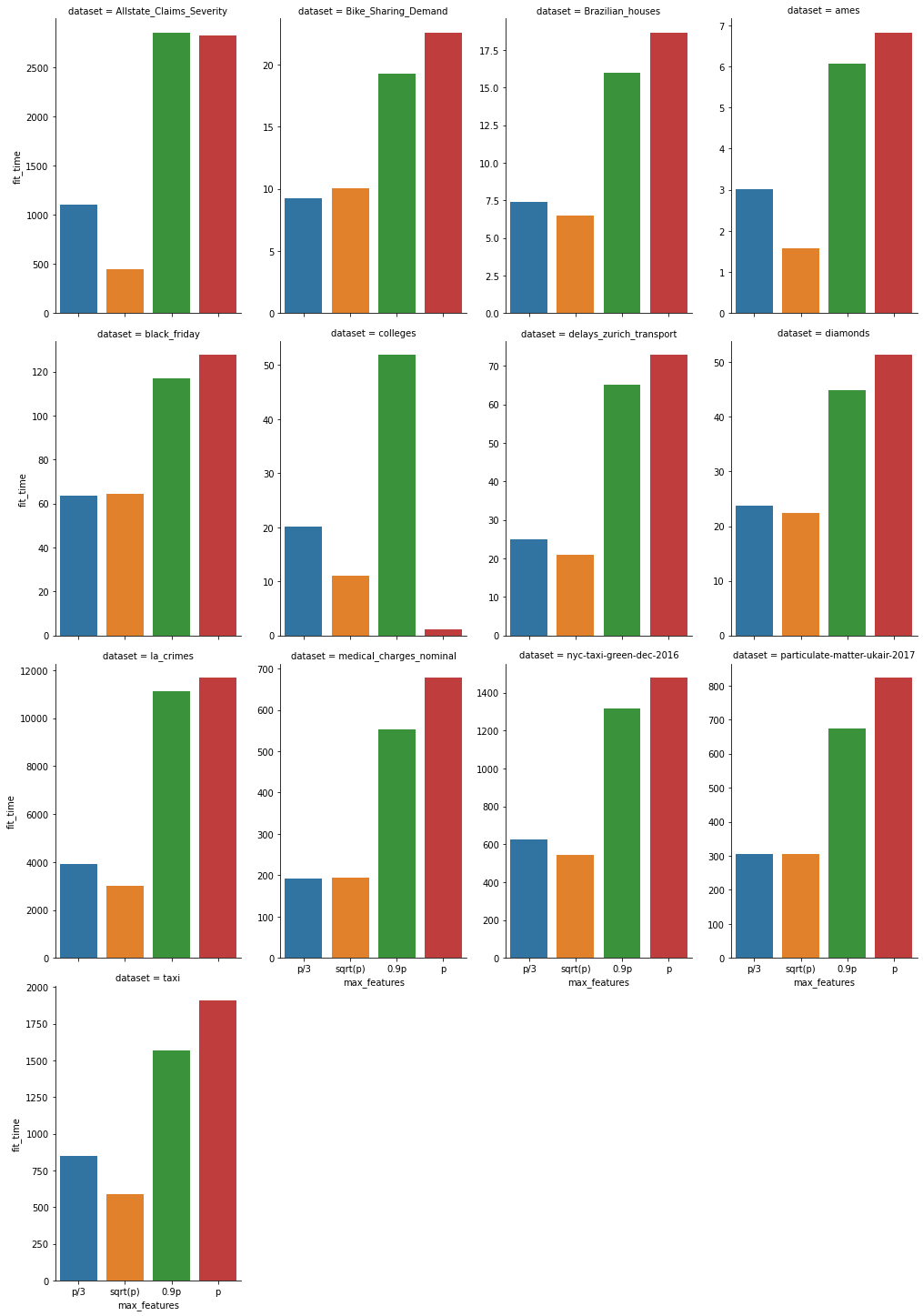
Full table in details.
we already allow floats so that’s equivalent to
max_features=.33
andmax_features=.9
: