Time-domain gating: Frequency-domain window required?
See original GitHub issueThis is a branch from the discussion on the mailing list: https://groups.google.com/g/scikit-rf/c/IoH8hNV58sI
Possibly related to issue #734.
The problem is with the roll-off in the edges of the frequency-domain results after gating (at f_min and f_max). A perfectly straight line is provided by PNA transforms. They probably apply a frequency-domain window before the gating.
Discussion: Is this required or advised to do by default?
The theoretical background is covered in an application note by Agilent/Keysight:
Example code based on the data by Roger in the mailing list:
from skrf.media.coaxial import Coaxial
import skrf
import matplotlib.pyplot as plt
import numpy as np
# reflection delays in nanoseconds
t1 = 758.725e-6
t2 = 82.759e-3
t3 = 416.759e-3
t4 = 508.759e-3
# window settings
freq = skrf.Frequency(0.05, 33, 1000, 'GHz')
print(freq.f)
window_type = ('tukey', 0.5)
t_center = t2
t_span = 0.25 # 150 ps
# create network
media = Coaxial(frequency=freq, z0=50)
coax_line_1 = media.line(0.5 * t2, 'ns', z0=50, embed=True)
coax_line_2 = media.line(0.5 * (t3 - t2), 'ns', z0=25, embed=True)
coax_line_3 = media.line(0.5 * t2, 'ns', z0=50, embed=True)
nw_total = coax_line_1 ** coax_line_2 ** coax_line_3
# apply gate
nw_gated = skrf.time_gate(nw_total.s11, window=window_type, center=t_center, span=t_span)
# plotting
fig, ax = plt.subplots(3, 1)
nw_total.s11.plot_s_time(ax=ax[0], label='Original')
nw_gated.s11.plot_s_time(ax=ax[0], label='Gated')
ax[0].set_xlim(-0.5, 1.5)
ax[0].legend(loc='upper right')
nw_total.s11.plot_z_time_step(ax=ax[1], label='Original')
nw_gated.s11.plot_z_time_step(ax=ax[1], label='Gated')
ax[1].set_xlim(-0.5, 1.5)
nw_total.s11.plot_s_mag(ax=ax[2], label='Original')
nw_gated.s11.plot_s_mag(ax=ax[2], label='Gated')
ax[2].set_ylim(0, 1)
plt.tight_layout()
plt.show()
With freq = skrf.Frequency(0.05, 33, 1000, 'GHz')
:
With freq = skrf.Frequency(0.05, 330, 1000, 'GHz')
:
Issue Analytics
- State:
- Created a year ago
- Comments:15 (1 by maintainers)
Top Results From Across the Web
Time Domain Gating - Copper Mountain Technologies
Time domain gating is a function that mathematically removes unwanted responses in the time domain. The function performs a time domain transformation, ...
Read more >Time Domain and Gating — scikit-rf Documentation
A major application of time-domain analysis is to use gating to isolate a single response in space. More information about the details of...
Read more >Advanced Antenna Measurement Techniques using Time ...
A vector response is measured between two antennas. • Time domain transformation is used on the frequency domain data. • Gating is used...
Read more >Time Domain - Keysight RFMW Sitemap
Window 4. On the original frequency measurement, turn Gating ON (Transform remains OFF). View the measurement without the effects of the two unwanted ......
Read more >Time Domain Measurements Using Vector Network Analyzers
The gating process can eliminate some of this necessary data that can introduce errors or ripple in the frequency domain response. As in....
Read more >
Top Related Medium Post
No results found
Top Related StackOverflow Question
No results found
Troubleshoot Live Code
Lightrun enables developers to add logs, metrics and snapshots to live code - no restarts or redeploys required.
Start Free
Top Related Reddit Thread
No results found
Top Related Hackernoon Post
No results found
Top Related Tweet
No results found
Top Related Dev.to Post
No results found
Top Related Hashnode Post
No results found
Keysight reference This document is excellent and I have studied it many times!!! Low-pass and band-pass You are absolutley correct to state that where you can you should use low-pass. I am always thinking in terms of band-pass because I work at such high frequencies. For me the mismatched line is unusual and something I am working on with NPL in the UK and it is in fact their data that you are using with their approval. Convolution or FTT They should be mathematically equivalent but to get the equivalence we have to treat the data correctly. With Roll-off
With no roll-off
It is easier to visualise why the convolution needs data beyond the last point because as the kernel starts to move past that point the signal will drop-off unless you pad out the data using Reflect or similar. We should always be careful to think about what the final points really mean in this case.
In the FFT case if we do not window the data will have a sharp transition at the edge as it will most likely not be zero. The window forces the data at the edge to go to zero. Then you need to remove it when you transform back.
Windowing in Frequency domain If you window in the frequency domain using Network.windowed before Fourier transforming to the time domain you have to divide the network by the window when you transform back to the frequency domain. So if you used the Network.windowed method with all the defaults you would have to divide by the equivalent window as follows:
I have tested this in the past and it works fine.
How to proceed My view would be:
Ok, things have been really confusing, but I think I sorted it out. Various different topics got mixed, all described very well in the application note by Agilent linked above:
irfft()
andrfft()
fromnumpy.fft
do exactly this.ifft()
andfft()
fromnumpy.fft
do exactly this.The current implementation of
time_gate()
performs time-domain band-pass mode. What we see is actually in line with the statements in Agilent’s application note linked above:Sorry for all the plots, but it’s really interesting to compare different setups. As you will see, the overall frequency span of the measurement and the (relative?) span of the window has a big impact. The type of window is not too important and only influences the ripple.
Setup 1:
freq = skrf.Frequency(0, 330, 1001, 'GHz')
Plot 1.1: comparing the time-domain responses using low-pass mode, band-pass mode, and the current skrf implementation (normal and windowed):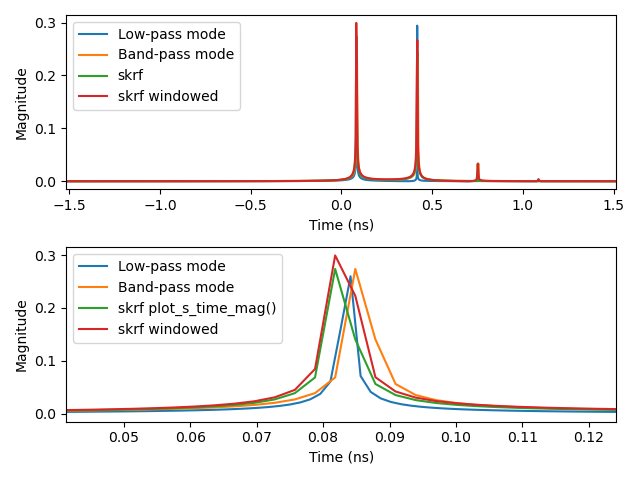
Plot 1.2: Gating the first reflection using low-pass and band-pass transforms with a Hann window: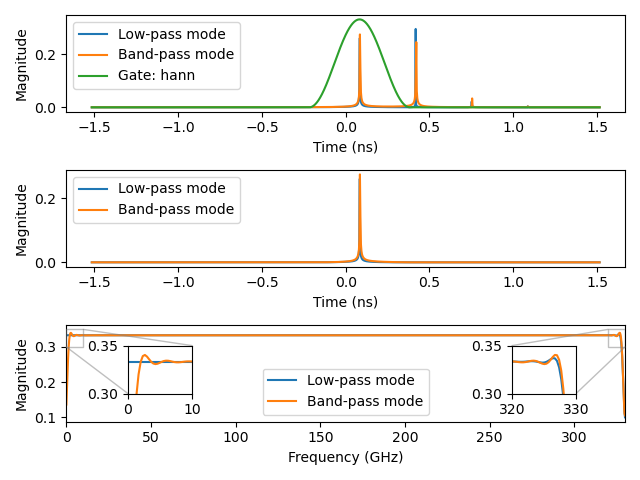
Plot 1.3: Gating the first reflection using low-pass and band-pass transforms with a Kaiser window (beta=10):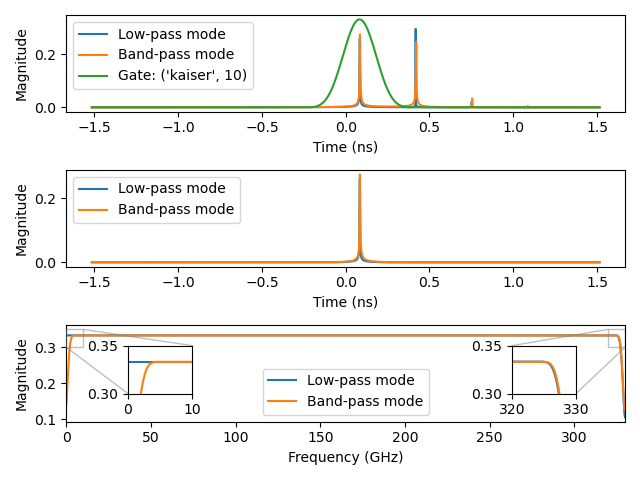
Plot 1.4: Gating the first reflection using low-pass and band-pass transforms with a Tukey window (alpha=0.6):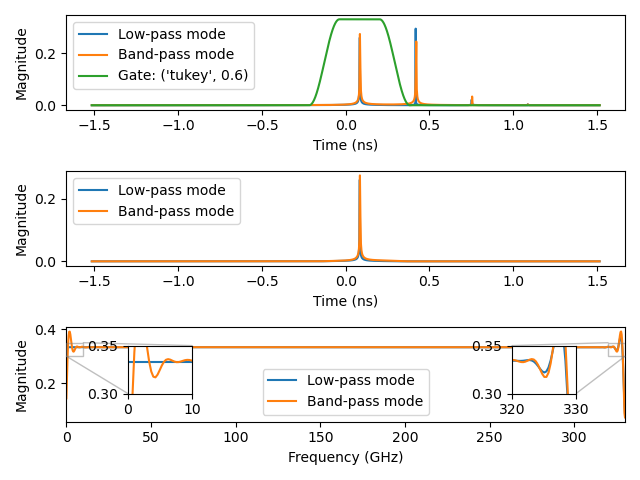
Setup 2:
freq = skrf.Frequency(0, 33, 1001, 'GHz')
Plot 2.1: comparing the time-domain responses using low-pass mode, band-pass mode, and the current skrf implementation (normal and windowed):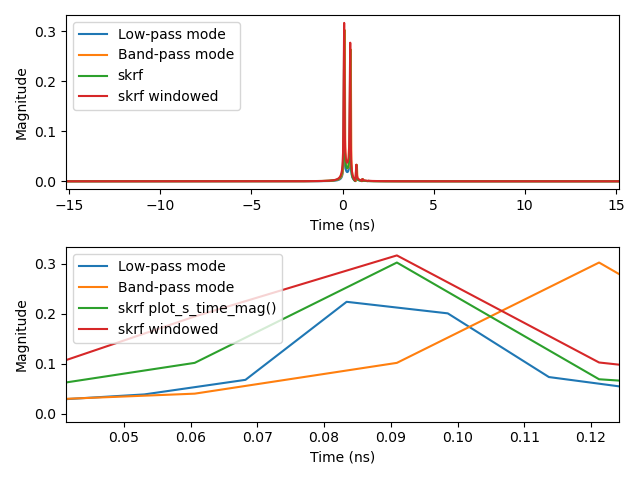
Plot 2.2: Gating the first reflection using low-pass and band-pass transforms with a Hann window: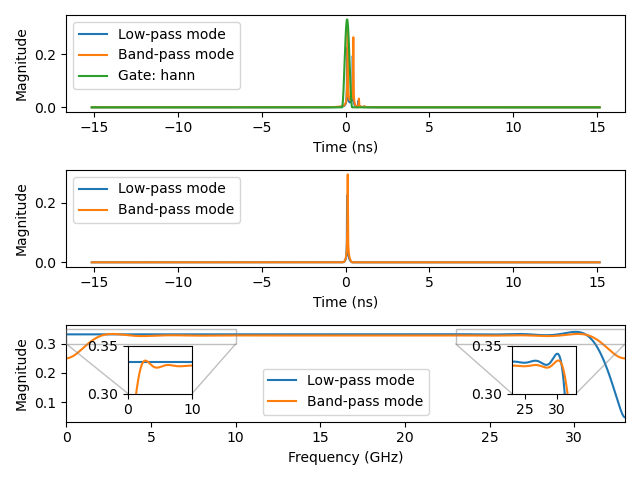
Plot 2.3: Gating the first reflection using low-pass and band-pass transforms with a Kaiser window (beta=10):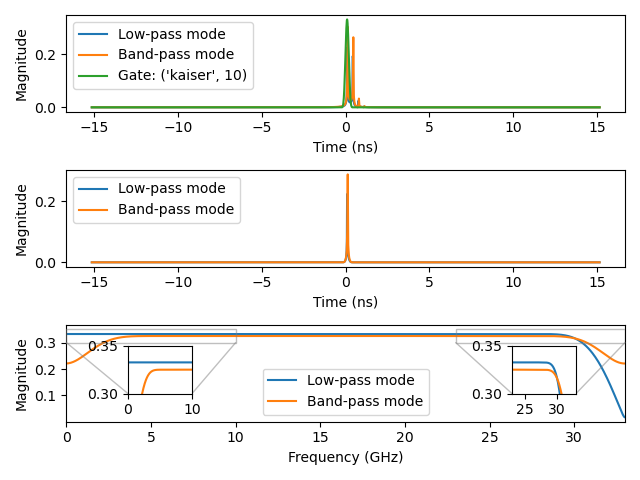
Plot 2.4: Gating the first reflection using low-pass and band-pass transforms with a Tukey window (alpha=0.6):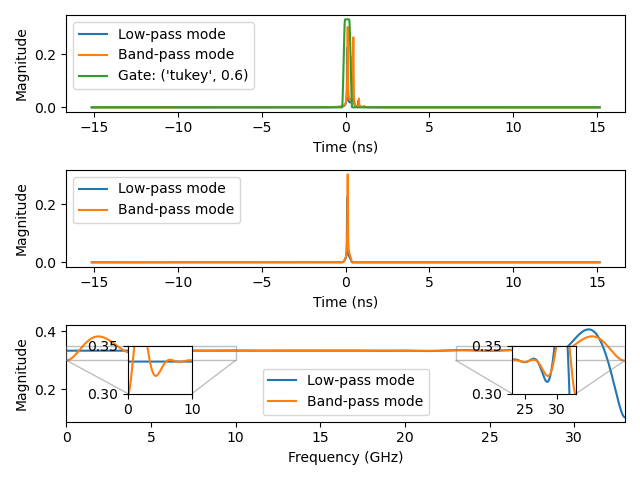
Setup 3:
freq = skrf.Frequency(0, 30, 1001, 'GHz')
Plot 3.1: comparing the time-domain responses using low-pass mode, band-pass mode, and the current skrf implementation (normal and windowed):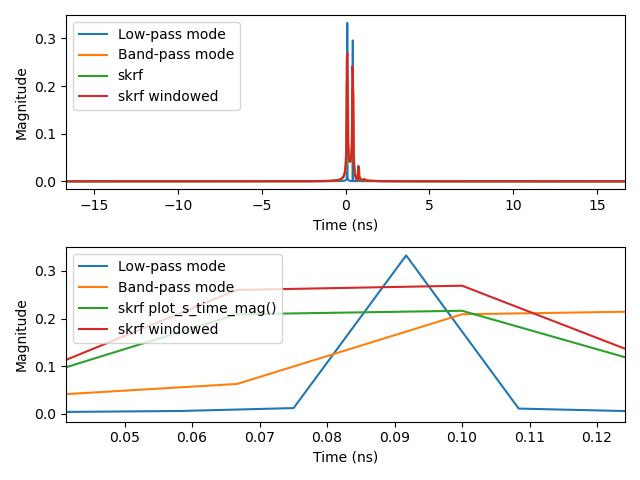
Plot 3.2: Gating the first reflection using low-pass and band-pass transforms with a Hann window: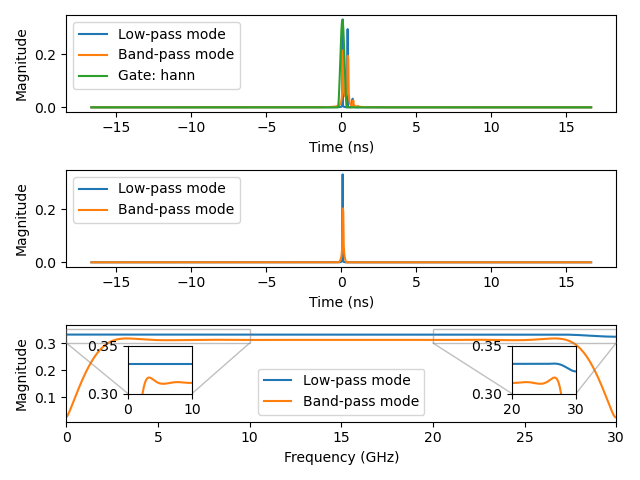
Plot 3.3: Gating the first reflection using low-pass and band-pass transforms with a Kaiser window (beta=10):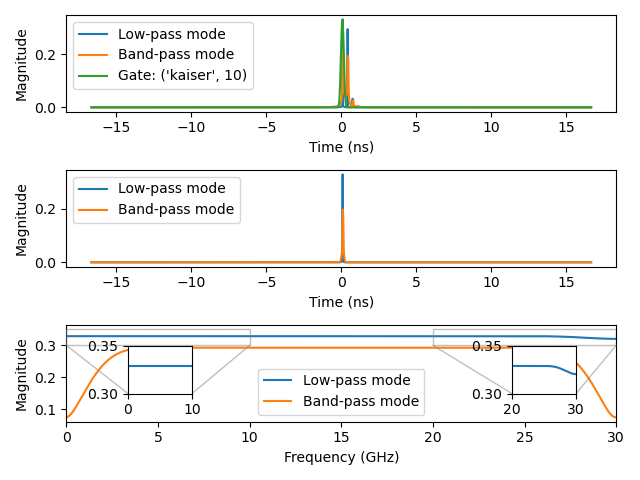
Plot 3.4: Gating the first reflection using low-pass and band-pass transforms with a Tukey window (alpha=0.6):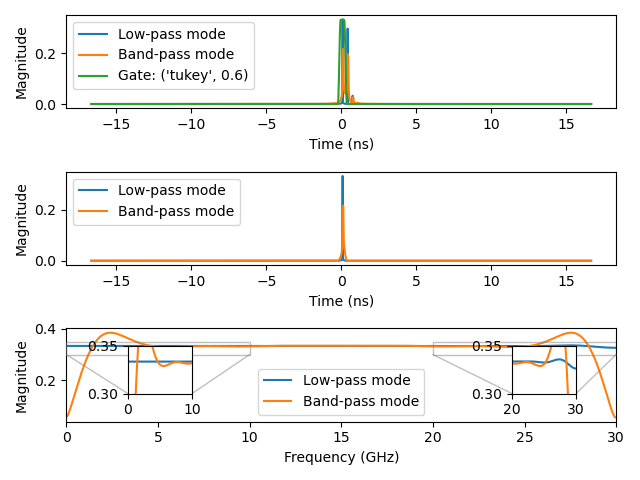